- Originally published in CFO Dive.
The Artificial Intelligence (AI) revolution seems to suggest that we should all become “cyborgs” in the brave new era of finance, wiping away all human interaction in our processes. So, should we mindlessly aspire to this vision in a zombie-like fashion, or is there a happy medium that might be more achievable and advantageous?
Finance is all about due diligence, so should we not do our due diligence on AI? Critical questions are in order—e.g., will the organization ever fully trust AI decisions? Is there institutional knowledge about history, culture, etc., that gives us humans an advantage over AI? Is our data ready for AI-enabled technology? Are our numbers too critical to risk hallucinations? Is there even a financial business case to implement AI across our finance models?
Walk Before We Run
It is hard to deny that there is finance power in AI—expediting our finance research work in credit and collections, conversing with internal and external customers and vendors, quickly resolving mismatches and short-pays to keep our processes touchless, and coding forecast algorithms that may offer insights we have not seen before. The wish list and subsequent use cases go on and on.
So, should we prioritize the use case with the most impact on savings, competitive advantage, and customer service? Seems intuitive but try again. How about the use case that checks your data boxes—clean data, secure data, easily formatted data, and abundant data, etc.? That is your highest priority use case! The rest is secondary. Our internal experiments with use cases have demonstrated that you can waste time, resources, money, and momentum trying to prove out a use case with data that is in disrepair or non-existent. Find your data first, then ask what you can do with it that would be valuable.
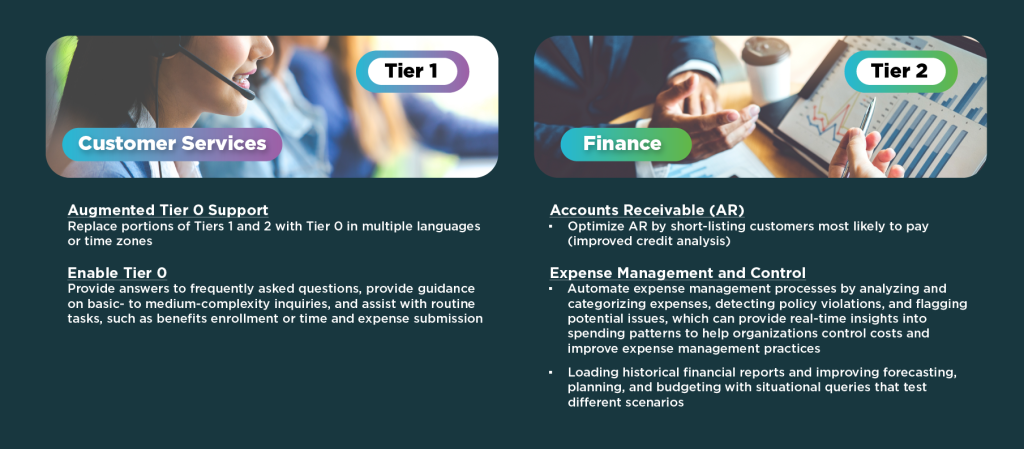
The data theme will become a common thread throughout your AI journey. It will expand beyond your first use case and inform how data should be organized, curated, secured, and governed. Work with your IT department to isolate the true source of data, determine how data will be managed (e.g., data warehouse/lake/lake house), what data will be used for (e.g., analytics, visualization, text content generation, or automation, etc.) and finally, what outputs are expected to be used (e.g., portals, reports, bots, or apps, etc.).
Data-Enabled AI Model
Chart a path for your data-enabled model as you learn from your first few use cases. Thinking through decision rights on data quality, security, standards, ownership, documentation, and oversight now will enable more use cases and save time struggling over these decisions when you are ready to operationalize use cases in a production environment.
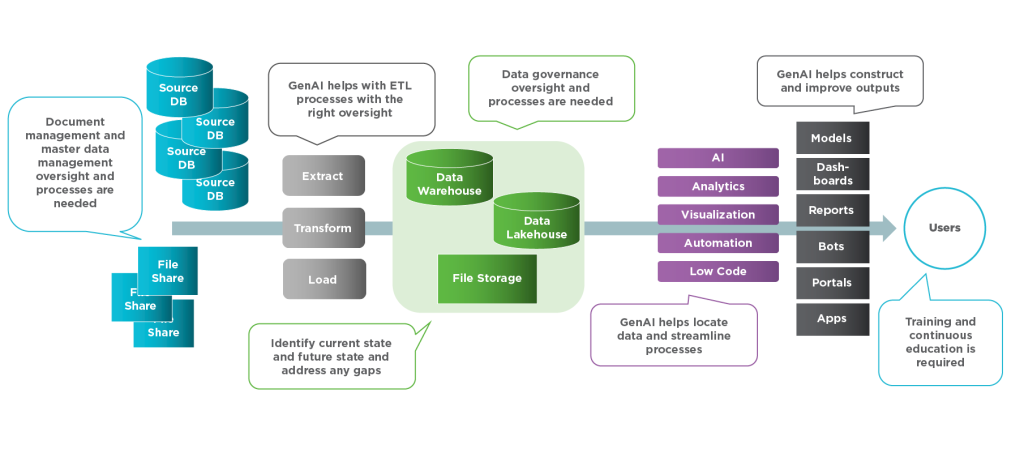
Key Data Governance Components
Data Quality Management:
- Regularly auditing data to identify and correct errors
- Implementing data validation rules to prevent the entry of invalid or incomplete data
- Establishing data cleansing processes to standardize and normalize data formats
Data Security and Privacy:
- Defining user roles and access controls to ensure that only authorized personnel can access sensitive data
- Implementing data encryption and secure storage mechanisms to protect data from unauthorized access or breaches
- Ensuring compliance with data privacy regulations
Data Policies and Standards:
- Developing and enforcing policies defining how data should be collected, stored, used, and shared within the organization
- Establishing data naming conventions to ensure consistency across systems
- Creating data retention and archival policies to manage the lifecycle of data assets
Data Ownership:
- Assigning data owners responsible for specific data domains’ quality, accuracy, and security
- Defining data stewards who oversee data management processes and ensure adherence to data governance policies
- Establishing clear roles and responsibilities for data-related tasks and decision-making
Data Documentation:
- Maintaining a data dictionary that defines data elements’ meanings, formats, and relationships
- Documenting data flows and transformations to provide transparency and traceability
- Tracking data lineage to understand data’s origin, usage, and impact across systems
Data Oversight:
- Forming a cross-functional team that includes representatives from IT, business units, legal, and compliance
- Regularly meeting to discuss data governance issues, review policies, and make data-related decisions
- Monitoring and reporting on data governance metrics to ensure continuous improvement
Adapting to AI Reality
If there is anything we learned from early automation days, it is that use cases are the easy part as compared to deploying new, and likely misunderstood, technology into production environments. This is particularly the case when there is any component of “citizen development,” which is highly likely with Generative AI solutions. Seemingly simple and impactful use cases can hit a “brick wall” of governance if appropriate parties have not been involved early and along the way. Internal control owners will need to understand the impacts of AI solutions on processes and sign off on risk mitigation. IT security must understand potential “leaks” that could jeopardize company data or intellectual property. The legal department will want to understand the risks of bias and hallucinations that may expose the company to litigation. Even the HR department may weigh in with potential job/role change risks, particularly in union environments.
The good news is that, hopefully, we have learned these lessons with new, innovative technology and citizen developer models. Scrutinizing use cases early on for touch points with other corporate departments is time well spent. Early education and constant involvement and communication will expedite the rollout. Many companies may have already developed AI governance models that can be leveraged with pre-defined decision rights that can apply to AI solutions.